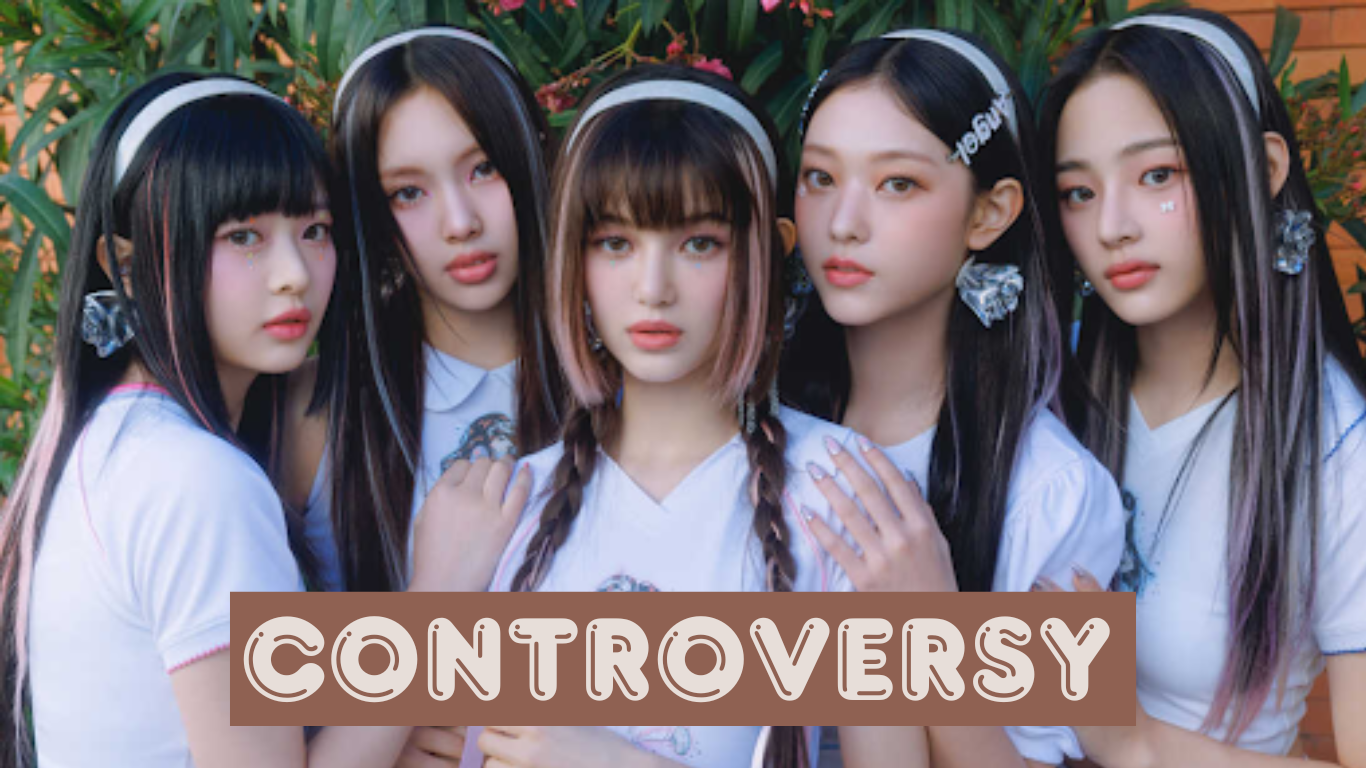
NewJeans controversy Shaking Up K-Pop NewJeans in the Eye of the Storm
NewJeans controversy The latest scandal rocking the K-pop industry involves NewJeans, a popular group, … Read more
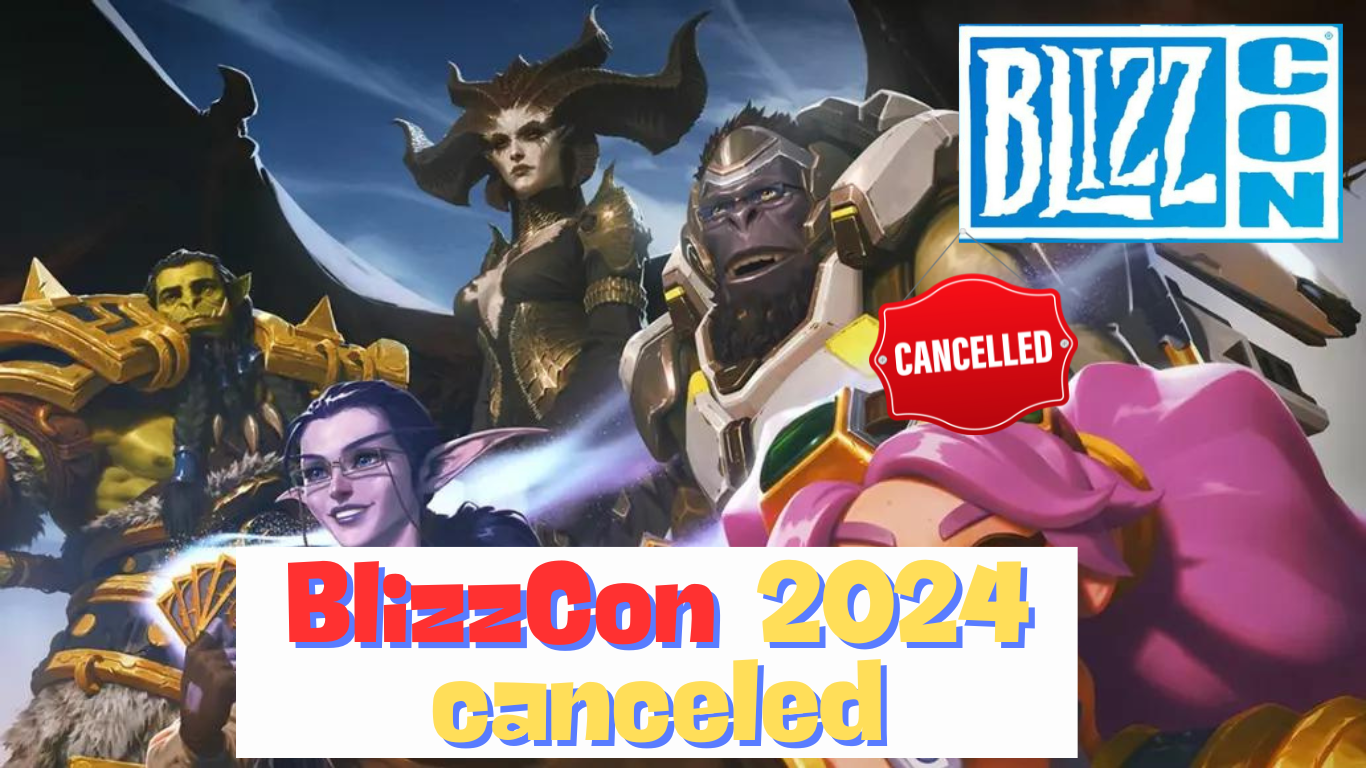
BlizzCon 2024 Canceled: Blizzard Announces Decision to Forego Annual Event
BlizzCon 2024 Canceled: Decision to Forego Annual Event Blizzard Entertainment, now under Microsoft’s ownership, … Read more
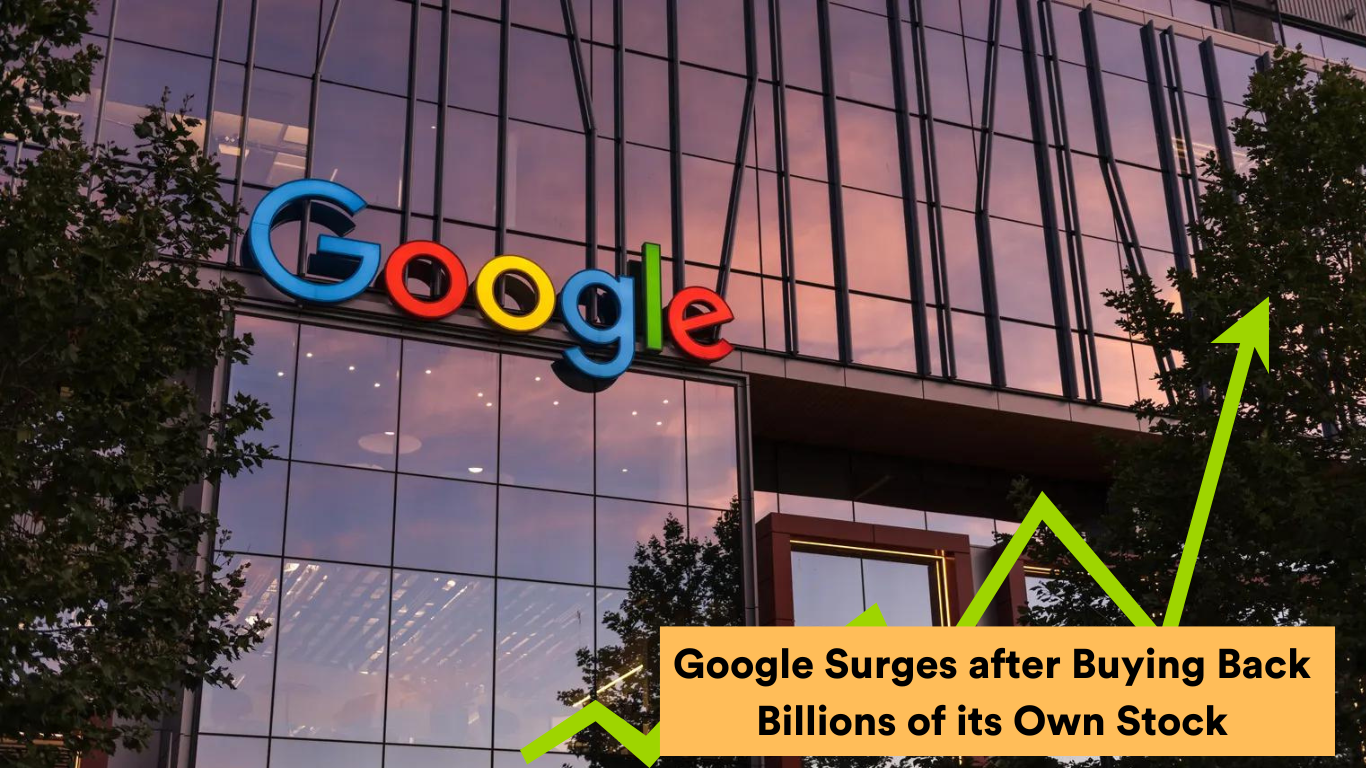
Google surges after buying back billions of dollars of its own stock
Google Surge: Alphabet, Snap, and Microsoft Lead the Way with Strong Quarterly Earnings Alphabet … Read more
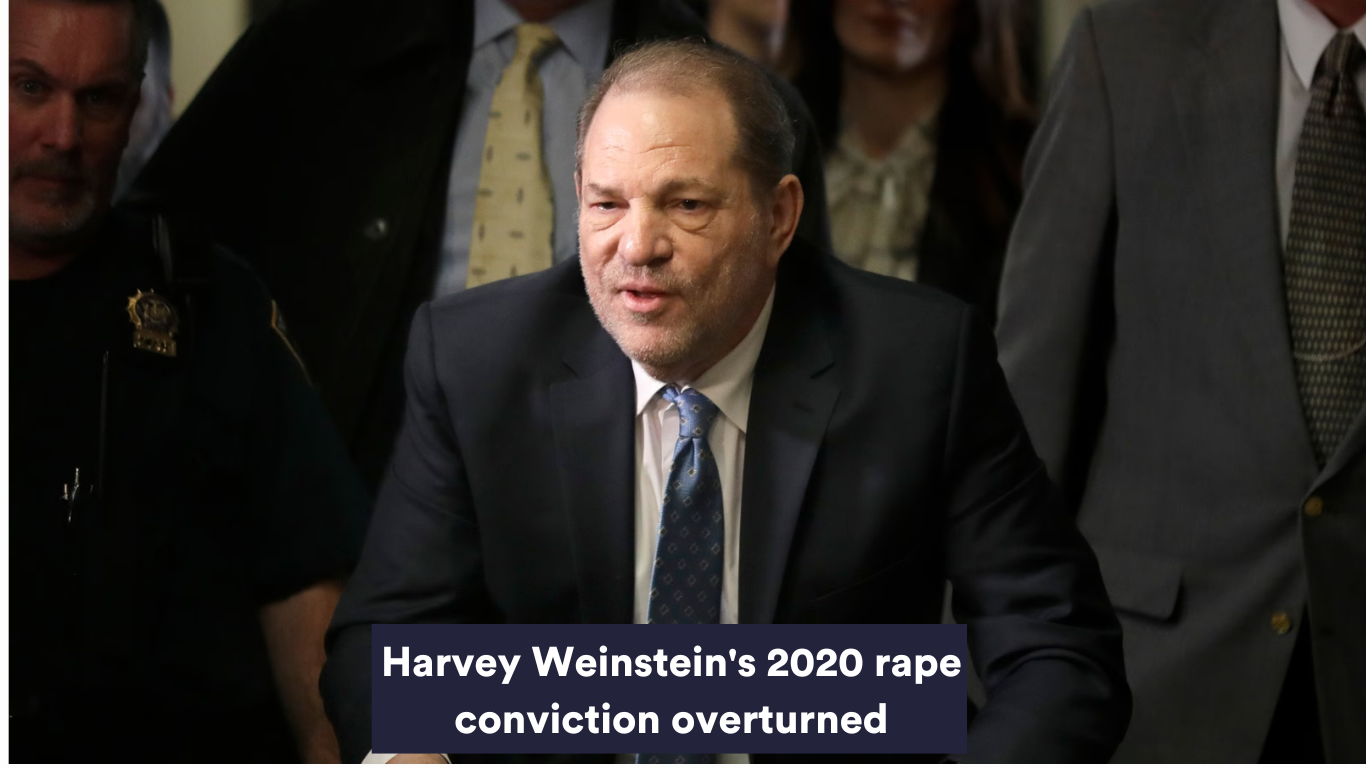
Harvey Weinstein’s 2020 Rape Conviction Overturned by High Court
Harvey Weinstein’s Conviction Overturned, Potential Retrial Looms The recent decision by a New York … Read more
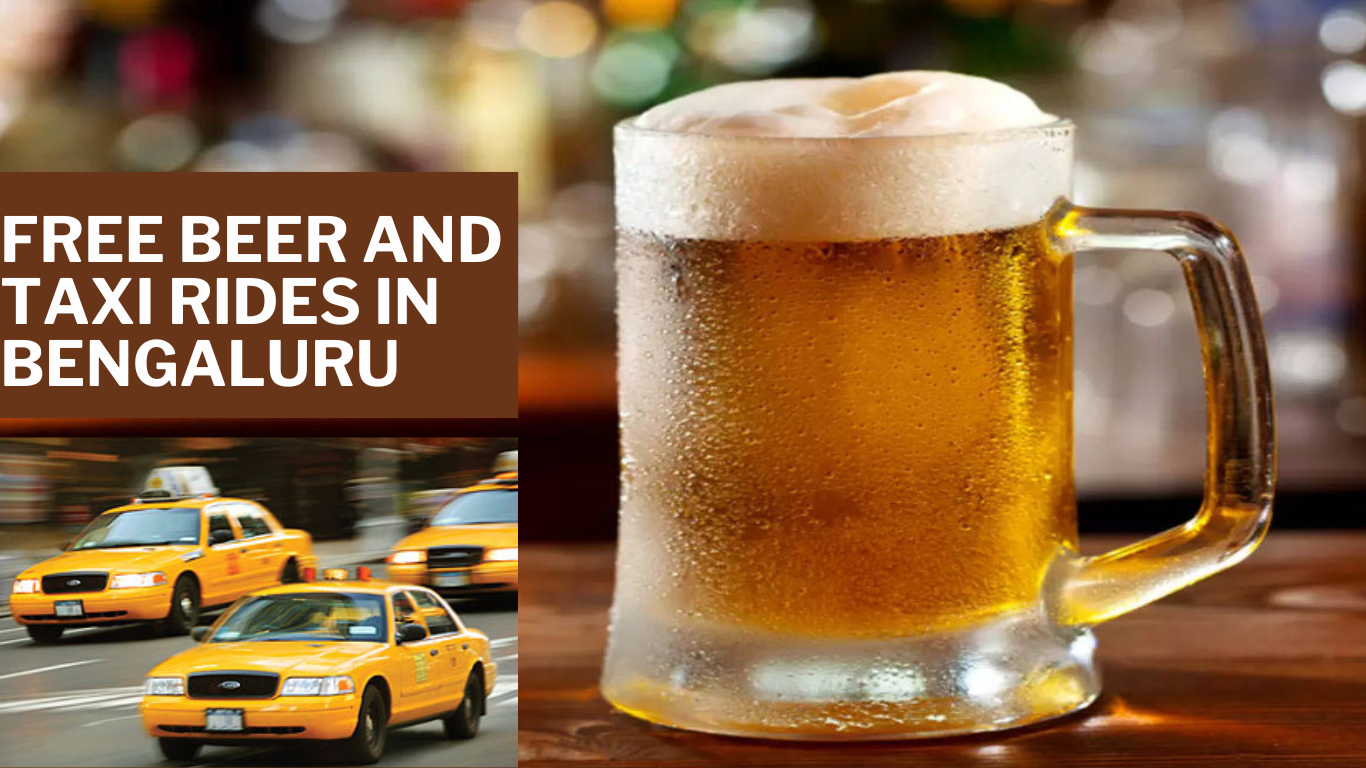
Bengaluru Businesses are Offering Free Beer, Food and Taxi Rides
Brief: Bengaluru businesses are offering incentives like discounted tickets, free beer, and reduced taxi … Read more
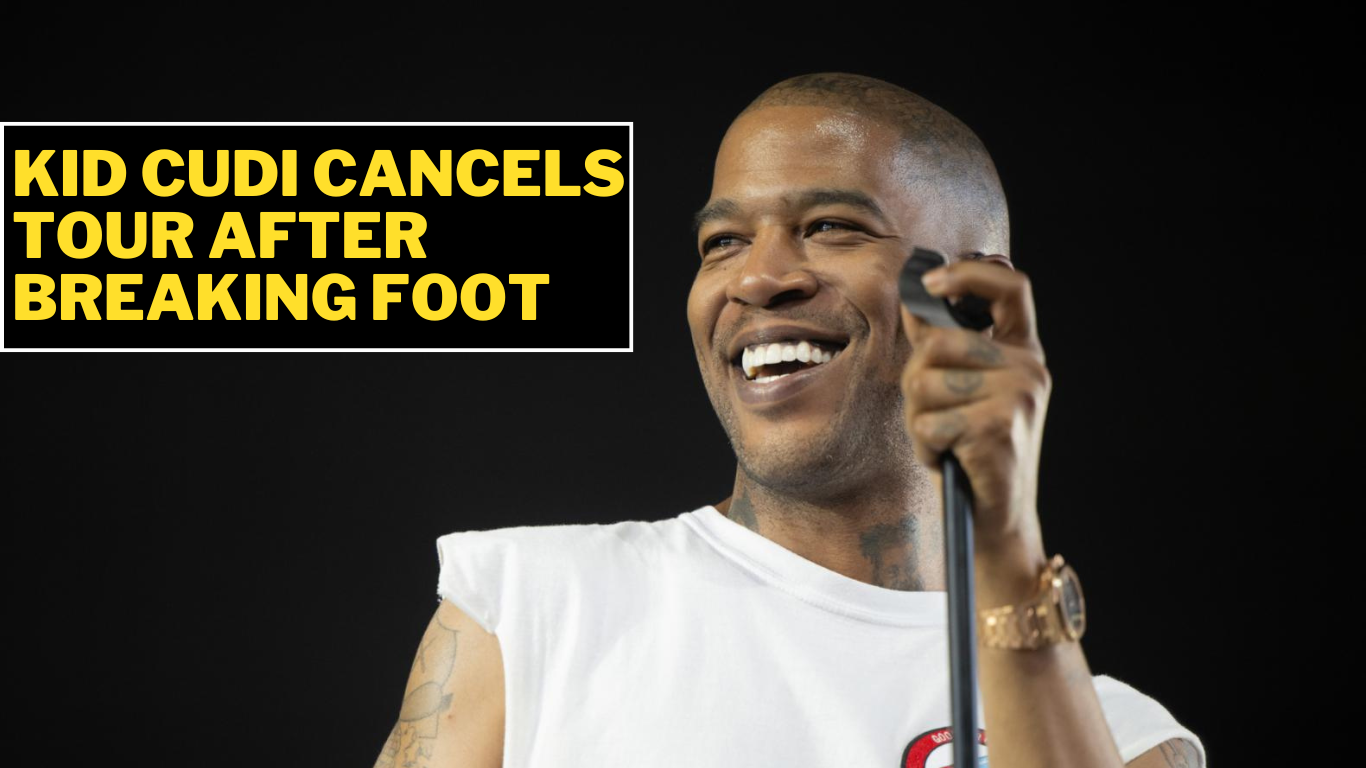
Kid Cudi Cancels Tour After Breaking Foot
Kid Cudi Cancels Tour: Fractures Foot at Coachella Rapper Kid Cudi cancels tour his … Read more
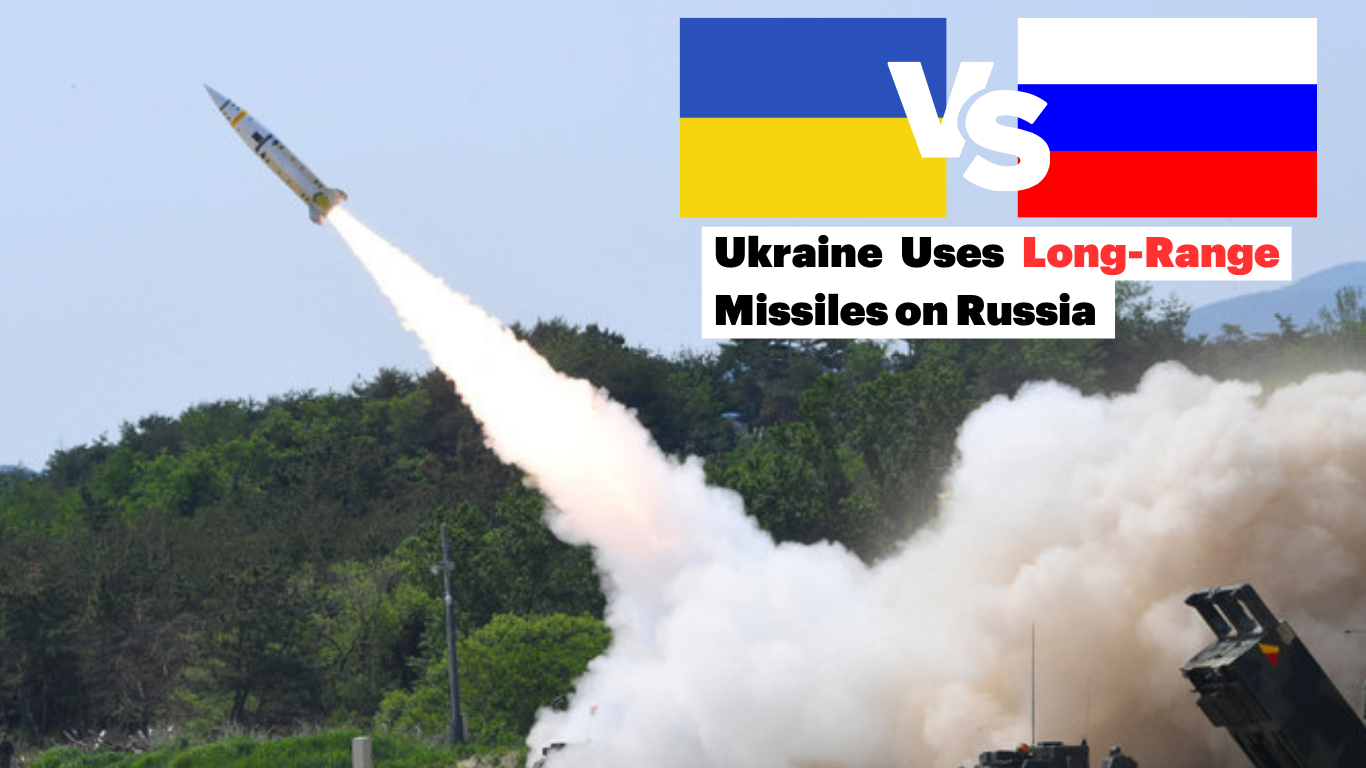
Ukraine Uses Long-Range Missiles On Russia secretly
Ukraine Uses Long-Range Missiles On Russia In a significant escalation of its military capabilities, … Read more
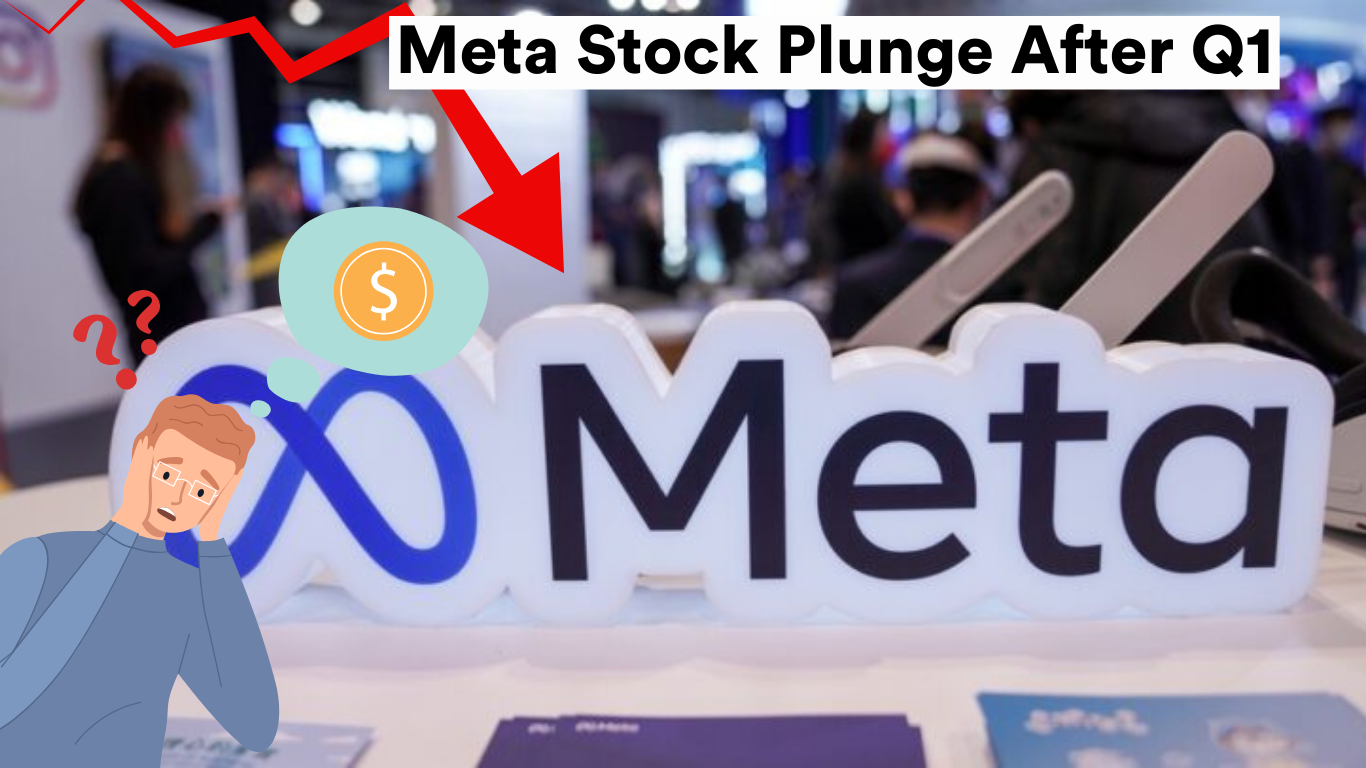
Meta Stock Fall by over 15% during after-hours trading on Wednesday
Brief: Meta Stock Fall by over 15% during after-hours trading on Wednesday. Meta delivered … Read more
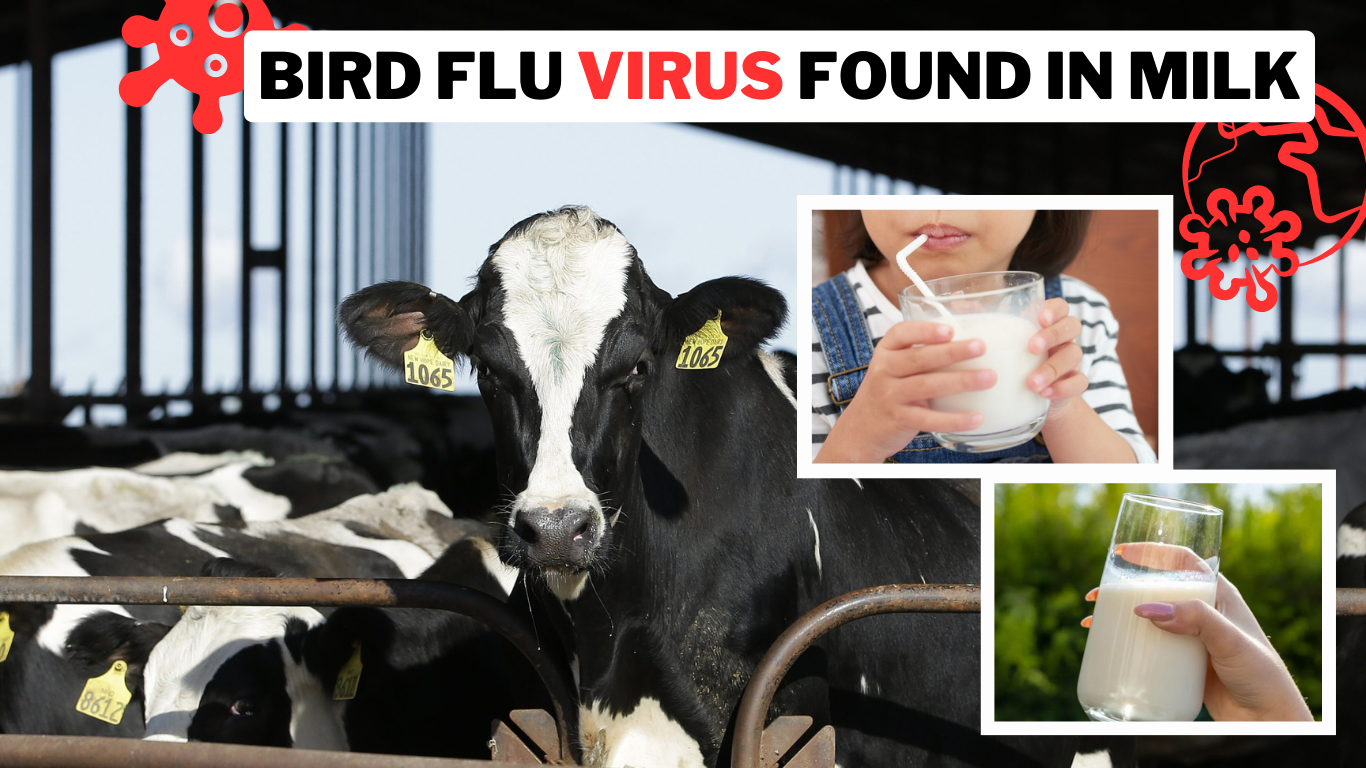
Bird Flu Virus Found in Milk: FDA Detects Traces in Pasteurized Samples
Bird Flu Virus Found in Milk The U.S. Food and Drug Administration (FDA) disclosed … Read more